Scaling ‘AI’ Offshore
Scaling ‘AI’ Offshore
Production-well serial data ticking in from electronically tagged downhole tools are now sorted, algorithmically, and in near real-time. While some are still testing AI’s backend (programming and hardware) and front-end (user controls and contextualized data) — without committing — operators in Norway are AI early adapters. Their engineer comms are already neural, just as young data scientists flush out the flaws, losses and some of the complexity in well tests.
By William Stoichevski
Comparative data goldmine: OKEA’s Draugen oil platform.
Image courtesy OKEANowhere offshore are the advantages of “AI” more impactful and the market-moving aspects clearer than during the “routine” well-test. Apart from engineers, geologists and company execs, even financial analysts are in on the scrutiny. We query the latter and find they have yet to consider whether AI production tools — whether “open API” or “closed” and cloud-based — make a difference in the bottom line. Perhaps they should.
Provided they have the right stuff, a producer, say, an indie, can “tweak” data models as they go on an “open” API system. Closed, cloud-based AI may be more regulatorily ready. Both can save millions of dollars during a year of well tests. Asked whether AI will become part of an oil company’s SGA costs, be crunched as COGM or be chalked up as R&D, our analyst is stumped. Offshore operators, too — despite being early AI movers — aren’t sure what to make of offshore AI.
“It’s like (doing) magic,” says Solution Seeker data scientist, Christine Foss-Sjulstad. “I think very many people think AI is a magical tool that fixes all your problems. So, getting customers in the mindset that they need some good data to start with is a tough discussion that we need to have. The more data you have, the better the product,” she tells Offshore Engineer on Teams. AI and “expectation management”, it seems, go hand-in-hand.
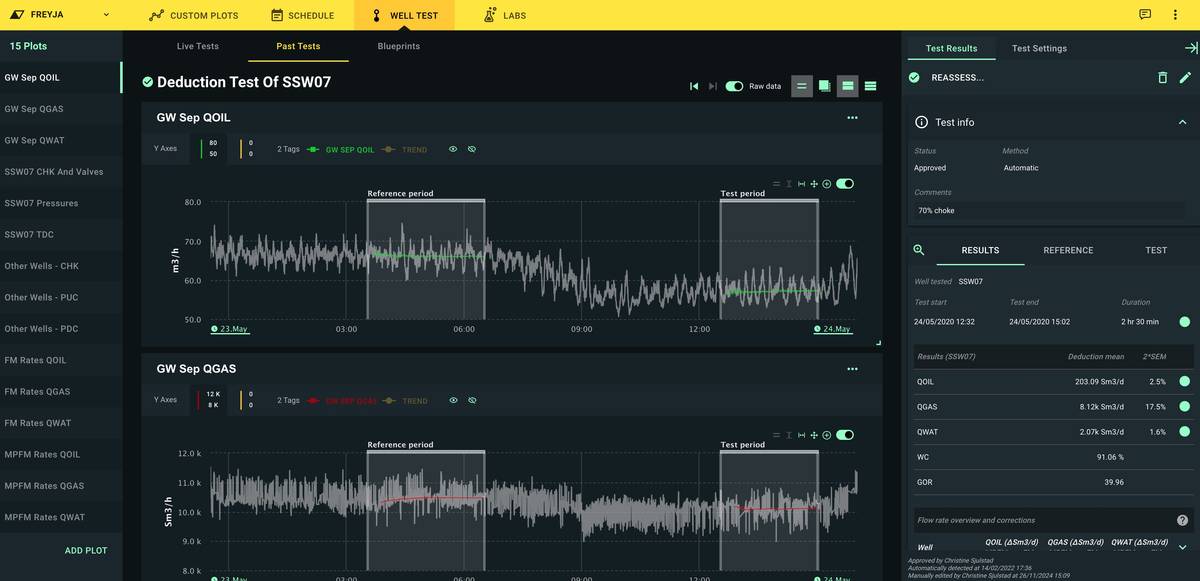
Solution Seeker
Their Web page suggests offshore workflows and efficiency measures can be reined in, digitally. Norway operator, OKEA, is using Solution Seeker’s Production Compass AI software and its data science tools at the Draugen field, where data-driven — and not just “physics-based” — flow-rate management is helping ensure better work flows and AI adaption elsewhere. Installed is a system of smart, “virtual-sensor” alarms that notify engineers when data models indicate noteworthy change, letting them other things. Draugen’s topside-controlled well tests, once laborious, are simpler for using Solution Seeker’s Well Test app. For its subsea wells, the app enables deduction testing that incurs minimal loss when shutting in one well at a time.
Stories about AI tend to be a mix of expectation and speculation about what AI should be able to do and where it is heading. Our analyst notices that offshore, service companies are pairing control systems with resident applications. “A lot of it refers to downhole data,” he remarks.
At ONS 2024, Solution Seeker promoted their Neural Compass Virtual Flow Meter, or VFM, with its data-driven methodology enabling timely reservoir and pressure estimates to add to its streamlined production test app and workflow monitoring, including comms with offshore engineers. To use the tools, producers need their own data “solidly structured”.
You can’t just place an algorithm on top of a mass of data, Foss-Sjulstad says. “It’s about contextualizing the data and triggering algorithms in a correct way,” so having data infrastructure in place is key for both producer and AI supplier.
Image courtesy Solution SeekerIt’s like (doing) magic. I think very many people think AI is a magical tool that fixes all your problems. So, getting customers in the mindset that they need some good data to start with is a tough discussion that we need to have. The more data you have, the better the product. - Christine Foss-Sjulstad, Data Scientist, Solution Seeker
Seeing Human Error
Gathering and interpreting client data sets are how Foss-Sjulstad and her overwhelmingly under-35 team of data scientists find solutions to challenges that range from oil-water remedial work to models that don’t reflect well tests.
Another challenge lies in showing the limits of physics-based production modelling. A data-driven offering appears to offer easy data control and model maintenance. Engineers’ fears should be eased by knowing Solution Seeker products and tech have since 2013 been developed in close collaboration with production experts.
Virtual flow metering is a “tricky and complex” field of technology. The key info is the well test, which Solution Seeker defines a good when “you’re actually approving tests and you have a very standardized workflow” while being able to easily import the well test data, other standard instrument data like pressure, temperature, choke sizes etc., when you’re “good to go”. Solution Seeker say they see human mistakes during well tests, as their tech autodetects well tests and provide info on “uncertainties” for different test results. “When we look at previous well tests, we see that there has been … a lot of human error. So, if it isn’t very intuitive, then you might not enter a certain time that perhaps you should have looked at to recognize that perhaps this wasn’t a really good well test,” Foss-Sjustad says.
When talking to Solution Seeker, it is difficult not to flash back to a time — not long ago — when Increased Oil Recovery and Enhanced Oil Recovery were all the rage, before cost-cutting became the thing. Solution Seeker, despite its software start-up vibe (it is an NTNU university spinoff), did, in fact, emerge out of that era (in 2013) and was created to answer all those needs. Their young vibe is real, however, although anchored in solid, grey-haired reservoir-flow and well-integrity experience.
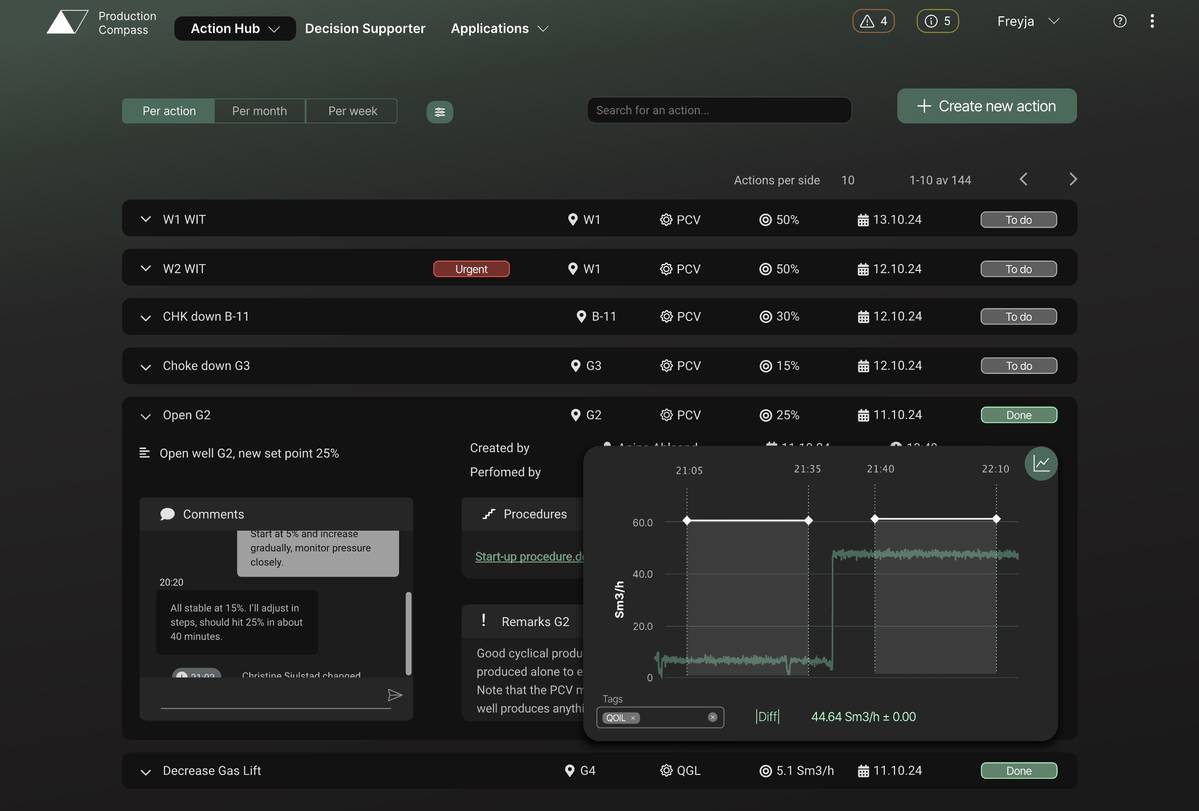
Deferred Production
The staggeringly wealth-generative and tech-heavy Norwegian oil industry is well onboard with Solution Seeker’s approach. Only Equinor and Svall are understood to not be using Solution Seeker tech among Norway’s operators.
While their current AI offering streamlines workflows and backs up physics-based production modelling, Solution Seeker are nevertheless upping their AI game and also adding use cases for machine-learning, at least with OKEA. Yet, it’s their well-test app that has convincingly won over a client list that’s the Who’s Who of hydrocarbons.
“The well-test app … is fairly off-the-shelf, standardized and works from the get-go,” Foss-Sjustad says, adding that deduction testing has also won over clients because “its crucial to know when you’re not getting enough information during the additional time when you’re shutting in a well. Being able to see the uncertainties and how they converge and be able to say, “Okay, now I can actually start up my well”.” Beyond the algorithm, visualization, can now end a test earlier than once thought correct, and at least one client is on the record saying they avoided deferred production worth about 6 million kroner (USD 600,000) per well test (they average 12 tests a year)! “It’s fairly simple. It creates value from the get-go.”
Solution Seeker’s recently released Neural Compass VFM app is used as both backup or as sole evaluator of flow. Some oil companies might also use a mechanistic MPFM which would have to be “fused” to it to add “information value”, making setting up “out-of-the-box” difficult and highlighting an industry with no “off-the-shelf” app to relay on for production. It doesn’t help that “Data infrastructure isn’t standardized across producing fields, (digital) platforms and different companies”. Custom set-ups, the fine-tuning of existing apps and the adding of new features “in-stride” appears to be the rule.
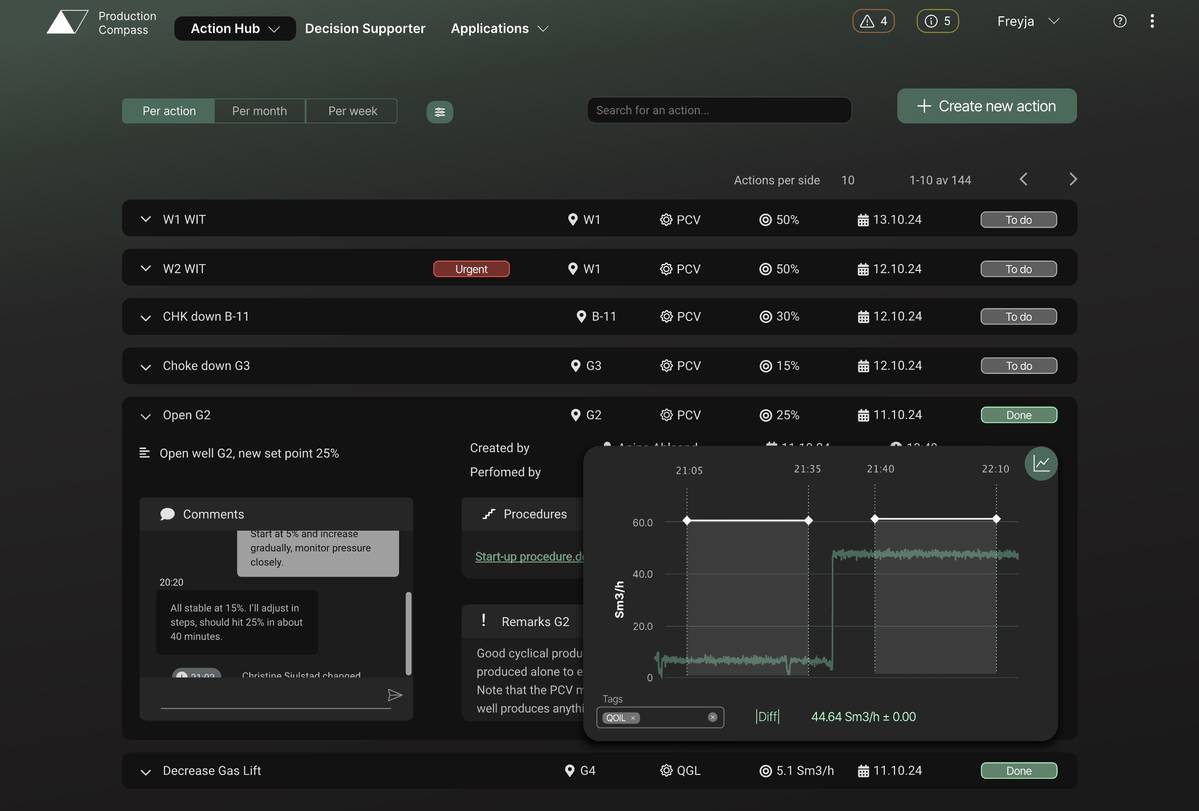
Training AI and Staff
Asked whether operator employees or the AI itself had to be trained, Foss-Sjulstad says, “It depends.”
“VFM (Virtual Field Manager) is a service we deliver mostly from our side, training and updating the model as well as deployment. What the client sees is an updated signal, like any other tag. Training enters the picture on products that are workflow based. For the well test app, you need to have a tutorial with the client… The same applies to a product we have (Neural Compass), where you create actions, and you can cooperate with the offshore engineer or the offshore operator first to discuss an action and then to communicate back when done. That’s when you need to train engineers.”
As everywhere, selling AI isn’t all smooth sailing. Gone are the fundraising rounds, the Norwegian Research Council funding and the help from known innovators. It’s hard, too, to convince proud engineers using trusted physics-based models to open up to data-driven production management, however sound.
That, too, “depends on the use case”.