AI IN THE MARINE INDUSTRY
By The Millron Group
Machine learning, also known as Artificial Intelligence, is divided into three categories: Regression based machine learning (estimating a given number, price of stock, etc.), Classification (given parameters, makes a decision) and Generative (output is a text, image or audio/video) are seen through the use of The Millron Groups use of their proprietary technologies and methodologies. These transform industries by allowing machines to perform tasks that would typically require human intelligence. These tasks include problem-solving, decision-making, learning from experience, understanding language, and recognizing patterns in vast amounts of data. AI systems use algorithms that guide computers to process and analyze data. Over time, these systems improve through techniques like machine learning and deep learning (a method in artificial intelligence that teaches computers to process data in a way that is inspired by the human brain), enabling them to adapt to new information and evolve their decision-making abilities.
First off, machine learning allows computers to learn from data without needing explicit programming for each task. It enables AI systems to analyze large sets of data, identify patterns, and make predictions or decisions based on what they’ve learned. Machine learning is categorized into several approaches. Supervised learning involves training AI models on labelled data, where each input is paired with a known output. Over time, the model learns to predict the correct output for new, unseen inputs. In unsupervised learning, the AI system uses data without labelled outputs, identifying hidden patterns, clusters, or relationships within the data, often revealing insights that weren’t previously known. Reinforcement learning, meanwhile, involves the AI system learning through trial and error. It receives feedback in the form of rewards or penalties for its actions, and gradually adjusts its strategy to maximize positive outcomes.
Secondly, deep learning, which is a subset of machine learning, uses neural networks similar to the structure of the human brain. These neural networks consist of layers of interconnected nodes (neurons) that can learn to recognize complex patterns in data. Deep learning is particularly effective for tasks like image recognition, speech processing, and natural language understanding. This capability is behind technologies such as facial recognition, autonomous vehicles, and advanced medical diagnostics.
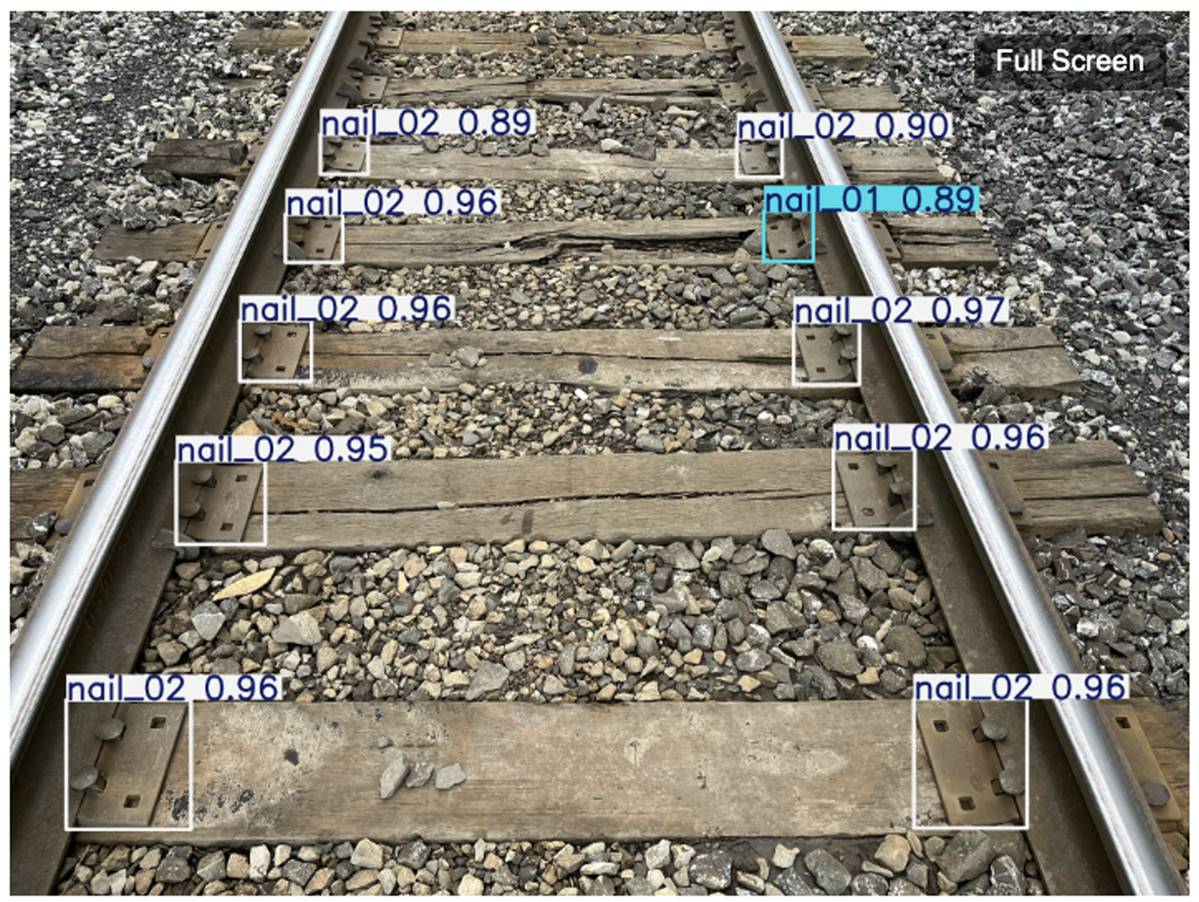
We also have something called Natural Language Processing. NLP enables AI systems to understand, interpret, and generate human language. It involves tasks like speech recognition, language translation, sentiment analysis, and chatbots. By analyzing the structure and meaning of text or spoken words, NLP allows AI systems to interact with people in a more natural, human-like manner.
One specific and commonly used AI in the market as of now is Computer Vision. This is the branch of AI that enables machines to interpret and understand visual data, such as images and videos. This is achieved through deep learning and pattern recognition techniques, allowing AI to identify objects, faces, or scenes in visual data. Applications of computer vision include facial recognition, automated image analysis in healthcare, and the vision systems in self-driving cars.
AI systems operate in a cycle of data collection, analysis, learning, and decision-making. AI systems rely on large datasets to learn and make decisions. These datasets can come from sensors, cameras, databases, or user inputs, depending on the application. The quality and quantity of data significantly affect the AI system’s performance. After collecting raw data, it is cleaned, structured, and transformed into a format that AI algorithms can understand. This step is crucial, as high-quality data ensures that the AI system makes accurate predictions and decisions.
AI algorithms analyze the processed data, identifying patterns or trends. These algorithms may include decision trees, neural networks, or support vector machines, depending on the complexity of the task. The algorithms process the data and make predictions or decisions based on identified patterns. A key feature of AI is its ability to learn from experience. After analyzing data and making decisions, AI systems improve by learning from the results. In reinforcement learning, for instance, the system adjusts its future actions based on rewards or penalties received for previous decisions. This continuous learning allows AI to become smarter and more accurate over time.
AI’s versatility has led to its adoption across numerous industries, enhancing automation, decision-making, and personalization. In healthcare, AI is revolutionizing healthcare by analyzing medical images, predicting disease outbreaks, and assisting in drug discovery. AI-powered diagnostic tools help doctors make more accurate decisions and deliver personalized treatment plans. In finance, AI helps detect fraudulent transactions, assess risks, and optimize investment strategies. By analyzing market data and consumer behavior, AI systems can forecast trends and identify opportunities for growth.
AI is increasingly part of our daily lives. Virtual assistants like Siri and Alexa use NLP to understand commands and provide assistance. Streaming platforms like Netflix and YouTube use AI to recommend content based on users' viewing history and preferences.
Similarly, e-commerce websites like Amazon leverage AI to personalize shopping experiences and suggest products. Autonomous vehicles, powered by AI, use sensors and computer vision to navigate roads, avoid obstacles, and make real-time decisions for safe driving. Autonomous vehicles rely heavily on deep learning and data analysis to improve their performance.
The marine industry, though vital to global trade, has traditionally relied on outdated, manual processes and legacy systems, particularly in areas like ship maintenance, cargo handling, and operational logistics. Many of these operations are reactive, slow, and inefficient, leading to unnecessary downtime, safety risks, and environmental impacts. AI offers a solution by modernizing these processes through predictive maintenance, real-time data monitoring, and automation, which optimize ship operations and ensure compliance with environmental and safety regulations. Importantly, AI doesn’t eliminate jobs but enhances them—by taking over repetitive, data-driven tasks, AI allows workers to focus on higher-level responsibilities, like decision-making and strategic planning. It also improves safety, reducing the need for dangerous manual inspections. Rather than replacing workers, AI tools help them be more effective, allowing for greater precision and efficiency in marine operations. Especially with the industry facing a shortage of marine personnel as well as the increasing complexity of the modern equipment that occupies the crew's time, AI is the umbrella that adds a layer of observation.
Such examples of AI implemented in the marine industry and elsewhere are seen with The Millron Group. Their predictive maintenance system uses machine learning to leverage real-time monitoring and predictive analytics which allows them to forecast equipment failures. This lets ship operators perform timely maintenance and prevent unplanned downtime. AI-driven operational optimization also helps automate tasks, manage resources, and make logistics more efficient. Another model is Millron RAILS which uses Computer Vision AI to accurately label and train its system for detecting rail anomalies through high-resolution image and video data. By using CVAT to annotate defects like cracks, wear, and other irregularities, the AI learns to recognize these patterns more effectively. This same technology can benefit the marine industry by training AI to identify damage on ships, docks, or marine infrastructure, helping to automate inspections, enhance maintenance, and improve safety through real-time monitoring and predictive maintenance. Other innovations like MAIA (Millron AI Assistant) uses the previously mentioned NLP AI to communicate and interact with employees and customers while their Winch and Motor Analyzer uses IoT and AI for problem diagnosis. All of these systems employed by The Millron Group use many of the different AI models described above to make these processes more efficient and safer.
Hopefully it can be seen that AI isn’t as complicated as it seems and has practical, real-world applications in the marine industry. AI technologies like predictive maintenance, operational optimization, and safety monitoring are already transforming the way ships and ports operate. Companies like The Millron Group makes these proprietary processes more efficient, safer and are leading the way, applying AI to improve efficiency, safety, and sustainability in marine operations, proving that AI is not just theoretical but a valuable tool for the future of the industry.